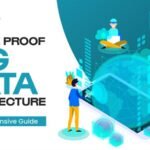
Future Proof Big Data Architecture: A Comprehensive Guide
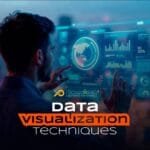
A Complete Guide To Data Visualization
Many organizations are sitting on a goldmine of information without realizing its true worth. Turning data into revenue isn't just a buzzword; it's a game-changing strategy that can transform your business.
From startups to large enterprises, ability to monetize data can open up new revenue streams, enhance customer experiences and give the business a competitive advantage. Let’s see how!!!
But where do you start? How do you navigate the complex landscape of data monetization without compromising privacy or ethics?
Here we are talking about the seven key strategies for turning data into revenue, from understanding data assets to building a data marketplace. Learn how to leverage partnerships, overcome challenges, and transform your data into a powerful revenue generator.
In this comprehensive guide, we'll explore seven key strategies for Turning Data into Revenue. From understanding your data assets to building a thriving data marketplace, we'll cover everything you need to know to unlock the hidden value in your information. Get ready to discover how you can leverage partnerships, overcome common challenges, and measure the success of your data monetization efforts. Let's dive in and explore how you can start transforming your data into a powerful revenue generator!
Understanding Your Data Assets
A. Identifying Valuable Data Types
When it comes to data monetization, not all data is created equal. Identifying the most valuable types of data is crucial for maximizing your revenue potential. Here are some of the most sought-after data types:
B. Assessing Data Quality and Relevance
Once you've identified valuable data types, it's crucial to assess their quality and relevance. High-quality, relevant data is more likely to generate revenue. Consider the following factors:
· Accuracy: Is the data free from errors and up-to-date?
· Completeness: Does the dataset have all necessary information?
· Consistency: Is the data uniform across different sources?
· Timeliness: How frequently is the data updated?
· Relevance: Does the data align with market demands?
C. Mapping Data To Potential Revenue Streams
With a clear understanding of your valuable data assets, the next step is to map them to potential revenue streams. This process involves:
1. Identifying industries or sectors that could benefit from your data
2. Analyzing market trends and demands
3. Exploring various monetization models (e.g., licensing, subscriptions, or data-as-a-service)
4. Assessing the potential ROI for each revenue stream
For Example: Companies like S&P Global generate 39% of their revenue from data subscriptions.
Direct Data Monetization Strategies
One of the most straightforward approaches to direct data monetization is to identify valuable datasets and offer them to potential buyers. Here's a breakdown of the process:
1. Identify valuable datasets
4. Find potential buyers
5. Establish secure data transfer methods
B. Creating and Marketing Data-Driven Reports
Adding value to raw data by providing insights and analysis is another effective strategy to monetize the raw data.
1. Identify trending topics or industry pain points
2. Analyze your data to extract relevant insights
3. Create visually appealing and informative reports
4. Develop a marketing strategy for your reports
5. Offer tiered pricing for different levels of detail
C. Offering Data-As-A-Service (Daas) Solutions
Data-as-a-service (DaaS) is an innovative approach to data monetization that provides on-demand access to data via cloud-based platforms.
For example, Weather.com sells weather data to airlines, agriculture, and logistics companies.
This model offers several advantages:
• Scalability: Easily accommodate growing data volumes and user bases
• Flexibility: Allow customers to access only the data they need
• Real-time updates: Provide the most current information to subscribers
Creating data-powered APIs allows other businesses to integrate your valuable data directly into their applications and services.
For Example: Platforms like Facebook and Google monetize user data through targeted advertising. In 2022, Google Ads generated $224.47 billion in revenue.
To succeed with API monetization:
1. Identify high-value data sets suitable for API access
2. Design user-friendly and well-documented APIs
3. Implement robust security measures
4. Offer tiered pricing models (e.g., freemium, pay-per-call, subscription)
5. Provide excellent developer support and resources
Indirect Data Monetization Approaches
A. Enhancing Existing Products with Data Insights
One of the most effective indirect data monetization approaches is enhancing existing products with data insights. By leveraging the wealth of information at your disposal, you can significantly improve your offerings, making them more valuable to customers and ultimately driving revenue growth.
For Example: Boeing uses Digital Twin technology to monitor aircraft performance, offering predictive maintenance services to airlines.
Here are some ways to enhance products using data insights:
• Predictive maintenance features
• Personalized recommendations
• Usage analytics for product optimization
• Real-time performance monitoring
For Example, Netflix uses data to recommend shows, driving 80% of its viewership.
Key personalization strategies include:
1. Customized product recommendations
2. Personalized marketing messages
3. Individualized pricing and offers
4. Tailored user interfaces and experiences
C. Optimizing Internal Processes for Cost Savings
Data-driven optimization of internal processes can lead to significant cost savings, indirectly contributing to revenue growth.
UPS uses real-time data from its ORION system to optimize delivery routes, saving $400
million annually.
Areas for process optimization:
• Supply chain management
• Resource allocation
• Inventory control
• Employee productivity
D. Strategic Decision-Making
By basing decisions on data-driven insights, companies can make more informed choices that lead to better outcomes and increased revenue.
For Example: Analyzing flight data to optimize routes and pricing, predict demand fluctuations, and schedule maintenance based on usage patterns.
Data can inform decisions in areas such as:
1. Market expansion
4. Investment opportunities
By implementing these indirect data monetization approaches, businesses can unlock the value of their data assets and drive revenue growth without directly selling their data.
Building a Data Marketplace
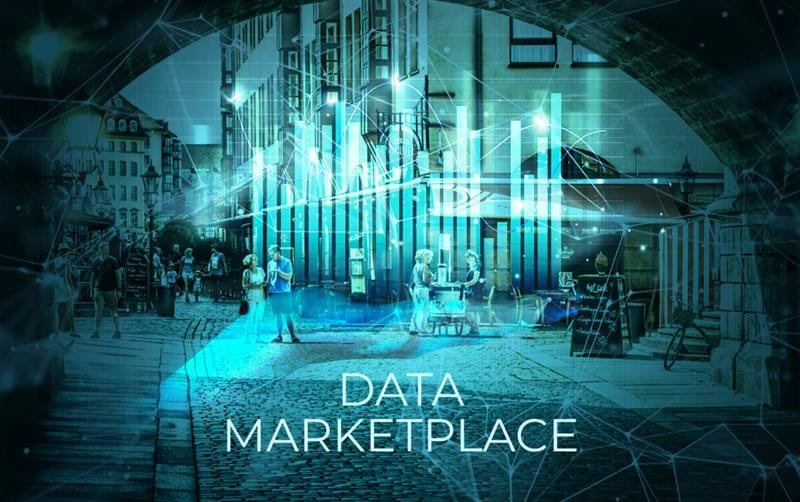
Data Marketplace
When building a data marketplace, selecting the appropriate platform or technology is crucial for success. Consider factors such as scalability, security features, and ease of use for both data providers and buyers. Popular options include cloud-based platforms like AWS Data Exchange or Google Cloud Marketplace, as well as specialized data marketplace solutions like Dawex or Snowflake Data Marketplace.
• Data anonymization and pseudonymization
• End-to-end encryption for data transfer
• Access controls and user authentication
• Regular security audits and penetration testing
C. Implementing Fair Pricing Models
Developing fair and transparent pricing models is essential for attracting both data providers and buyers. Consider the following approaches:
1. Subscription-based pricing for ongoing access
2. Pay-per-use models for specific data sets
3. Tiered pricing based on data quality or volume
4. Revenue-sharing agreements with data providers
D. Attracting and Retaining Data Buyers
To build a thriving data marketplace, focus on attracting and retaining quality data buyers. Strategies include:
• Offering diverse, high-quality data sets
• Providing excellent customer support and documentation
• Implementing a user-friendly interface for data discovery and purchase
• Developing a loyalty program for frequent buyers
By carefully considering these aspects, you can create a robust data marketplace that drives revenue and provides value to all participants.
Leveraging Partnerships for Data Monetization
A. Identifying Potential Data-Sharing Partners
For Example: Tesla’s self-driving cars collect vast amounts of data, which is used to improve autonomous driving algorithms. This data is also monetized through partnerships with other automakers. Here’s how real time data life cycle looks like.
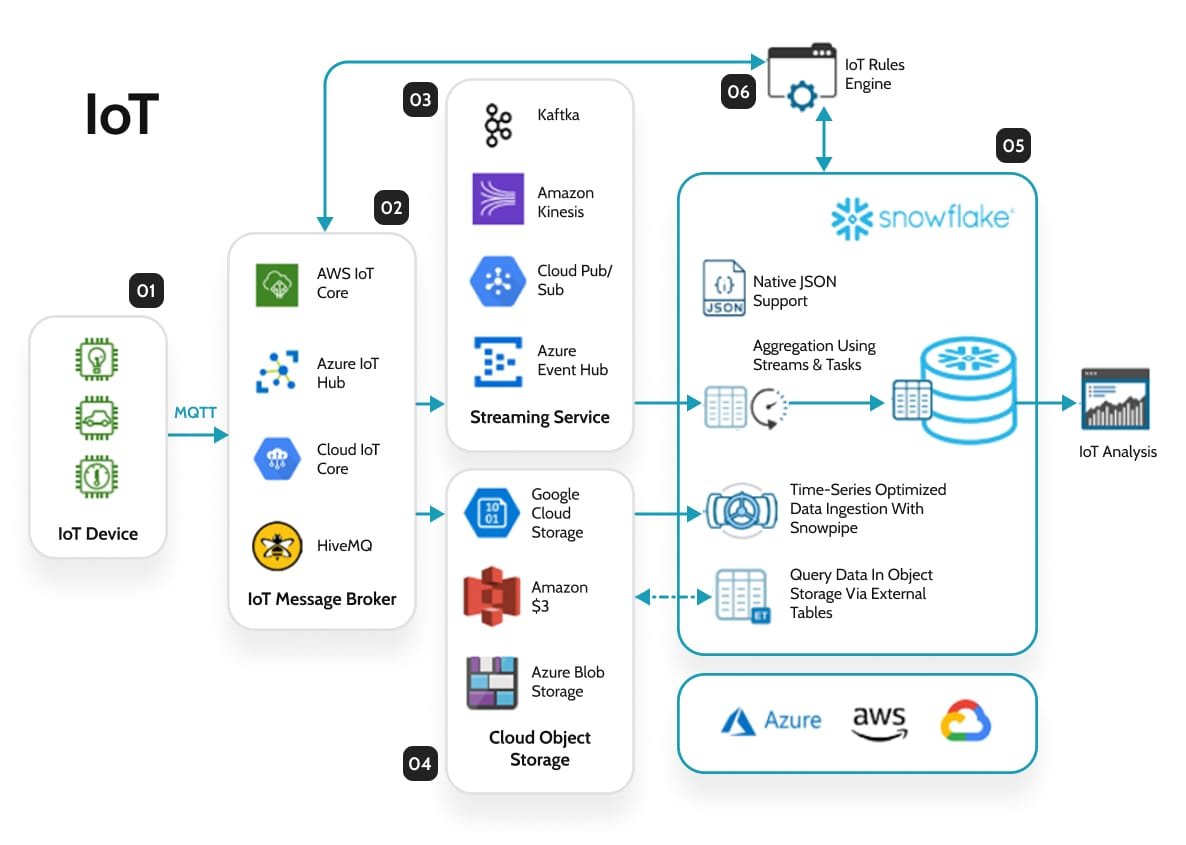
Identifying Potential Data-Sharing Partners
1. Complementary businesses
2. Industry leaders
3. Research institutions
4. Technology providers
5. Government agencies
• Data ownership and usage rights
• Revenue sharing models
• Data security and privacy measures
• Exclusivity clauses
• Duration of the partnership
C. Co-Creating Data-Driven Products or Services
With partnerships established, collaborate on co-creating data-driven products or services. This process involves:
1. Identifying market needs
2. Combining data assets strategically
3. Developing innovative solutions
4. Testing and refining offerings
5. Launching and marketing jointly
Relationships and Partnerships are the way to go in future. This collaborative approach not only maximizes the potential of existing data assets but also opens doors to new opportunities and insights.
Overcoming Data Monetization Challenges
A. Addressing Data Privacy Concerns
When monetizing data, privacy concerns are important. Organizations must implement robust data protection measures to safeguard sensitive information and maintain customer trust.
• Offer opt-out options for customers
Compliance with data protection regulations is crucial for successful data monetization. Failure to comply can result in hefty fines and reputational damage.
C. Managing Data Quality And Consistency
Poor data quality costs businesses an estimated $12.9 million annually.
High-quality, consistent data is essential for effective monetization. Poor data quality can lead to incorrect insights and diminished value.
• Implement data cleansing and validation processes
• Establish data governance frameworks
• Regularly audit and update data assets
• Use machine learning for anomaly detection
D. Dealing with Data Silos and Integration Issues
Data silos can hinder monetization efforts by preventing a holistic view of available data assets. Integrating disparate data sources is crucial for maximizing value.
Now that we've addressed key challenges, let's explore how to measure the success of your data monetization initiatives.
Future of Data Monetization
The future lies in generative AI and real-time data processing. Generative AI can create synthetic data, enabling businesses to train models without compromising privacy. Additionally, the rise of IoT and 5G will further accelerate data generation,
opening new avenues for monetization.
Conclusion
However, success requires a clear understanding of the Data Paradox: while data is abundant, its true value lies in how effectively it is managed and utilized. As Seth aptly puts it, "The better organizations get at dealing with data, the more value they will be able to generate from their AI efforts."
Interested in intellectual conversation on the topic?? Please feel free to reach out.
Guidelines for Creating Images
1. Data Monetization Workflow:
o Alt Text: "Workflow showing data collection, processing, analysis, and monetization."
o Description: A flowchart illustrating the steps from data collection to revenue generation.
3 Step Image
o Collection Sources
1. Internet: Transaction Data, Emails, Internet Content
2. Social Media: Posts, Comments, Images, and Videos
3. Sensors and Devices: IoT Data, Digital Footprint, AI & Synthetic Data
o Data Processing & Analysis: Ref Image.
o Monetization Image: Link to Impart step on the above image
A. Selling raw data
B. Creating and marketing data-driven reports
C. Offering data-as-a-service (DaaS) solutions
D. Developing data-powered APIs
B. Improving customer experiences through personalization
C. Optimizing internal processes for cost savings
D. Strategic decision-making
E. Building a Data Marketplace
2. Real-Time Data Processing:
o Alt Text: "Real-time data processing pipeline with IoT devices, cloud storage, and analytics tools."
o Description: A diagram showing how IoT devices send data to the cloud, where it is processed and analyzed in real time.
3. Generative AI in Data Monetization:
o Alt Text: "Generative AI creating synthetic data for predictive analytics."
o Description: An image depicting how generative AI models generate synthetic data for training predictive models.
o Ref Image
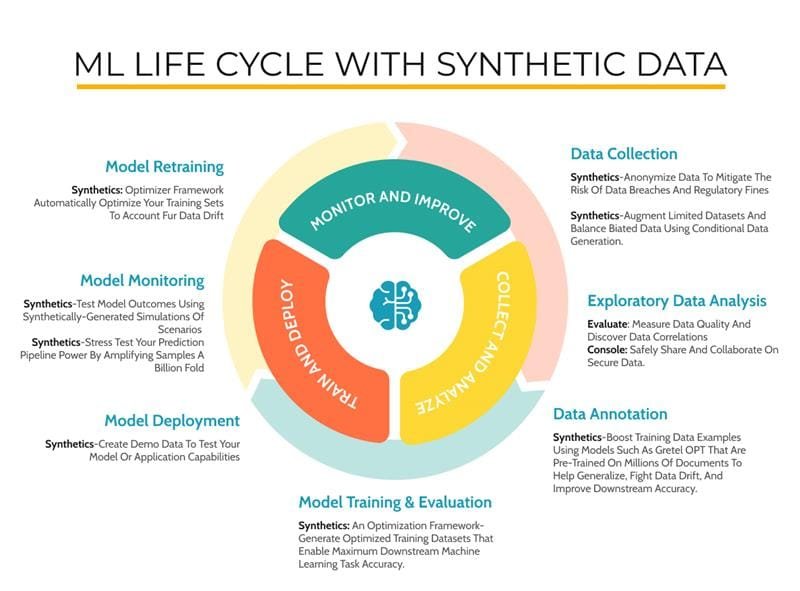
ML Life Cycle With Synthetic Data
This Article is Also here